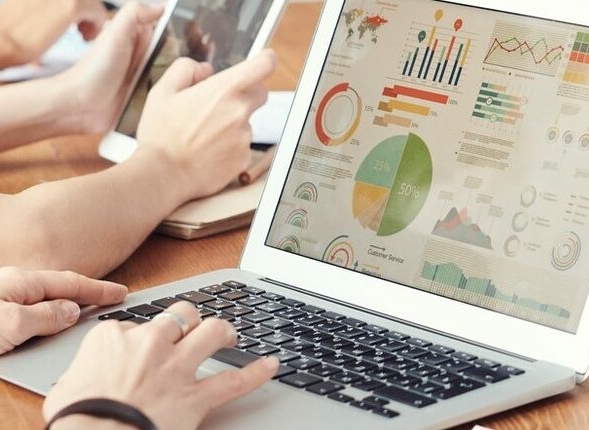
You’ve run your survey, have your data and now it is time to make sense of the information you have collected and understand what the data is telling you.
Start your analysis early
Considering the analysis at all stages in the research process means it is much easier to make sense of your findings. For example, earlier in the series we discussed the importance of planning your study in terms of scale e.g. talking to enough of the right people / employers so you can be confident in the accuracy of your results. We also advised that questions are written in such a way that enables easy interpretation of the answer.
Once the survey is underway you should be able to look at question summaries on survey platforms like Survey Monkey, which will indicate the direction and strength of findings. You can use these to:
- Check data quality: At some questions you might have ‘don’t know’ answer options, but if these attract too high a level of response, you’re not going to get much definitive insight. If this is the case, you should consider re-wording your question and adjusting your answer options.
Similarly, if you have allowed an ‘Other (specify)’ response option to closed questions, you should check how often this is being used. Remember that if you have a lot of free text answers, you’re going to need to manually group these into themes which will be a time-consuming process. It’s worth scanning through these at an early stage during the fieldwork period to see if you can add an extra pre-defined response option to save more work further down the line.
- Discuss emerging / interim findings: Looking at results early can also give you a head start on analysis and identify themes that are developing strongly. This will help set an overall framework for you to fill in with more detail later and help shape more formal analysis.
- Inform your more formal / detailed analysis outputs: If you have the ability to produce data outputs like tabulations in-house then looking at question summaries early on can help shape your final data-set. A look through early findings might identify a group of interest you might have not previously considered. For example, in a survey about first semester satisfaction and likelihood to drop out, one question might report a high level of dissatisfaction among students with how their course is delivered. It would be a good idea to track these students though the survey and compare their answers to other questions against those who are satisfied with course delivery to try and explain why they are dissatisfied.
Top tip: Try and make your data as user friendly as possible to minimise extra data cleaning at the end which is likely to distract you from actually interpreting and understanding what the data is telling you
Organising your final data set
You’ll want to organise your data so it doesn’t feel overwhelming, but also so it allows you to do some more detailed analysis. As well as looking at question summaries / frequencies, you’re likely to want to understand how answers differ depending on the type of (prospective) student, graduate or employer.
Sticking with the course satisfaction example. As well as understanding the total percentage of students likely to drop out, you’ll want to understand how this sentiment varies by different types of student based on characteristics like their gender or ethnicity, whether they are a home / international student etc.
You will also want to understand whether there is a specific type of student driving the overall finding. For example, if a high proportion of your survey responses are from international students who are more likely to say they want to drop out, then the overall figure reporting that are likely to drop out will also be high.
Running cross tabulations – crossing each question against different groups of interest is an effective way of organising your data to do this sort of analysis. On platforms like Survey Monkey, it is possible to filter results by certain groups or compare groups by questions and present this in cross-tabulations. If you’re able to export your survey data into Excel, then you can also replicate these sorts of outputs using pivot tables.
If you have the skills in-house to tabulate your data, you may find the following pointers useful:
- For questions that use rating scales, think about how to summarise this data, for example, creating a summary row of ‘agree’/‘strongly agree’ grouped together and ‘disagree’/’strongly disagree’ grouped together
- Think about combining certain questions in one data table, where a series of questions will be more compelling to report if combined. For example, a question capturing levels of loneliness with another that captures likelihood to drop out of their course.
- For numerical questions, think about showing the average to avoid having to manually calculate this at the analysis stage
Top tip: Remind yourself of the research aims and objectives when organising your data. This will help focus your mind and ensure you have all the data you need to answer the key questions
Interpreting data
The best way to conduct quantitative analysis is by taking a methodical approach and where possible, involving at least one other person so you can talk through your respective interpretations of the findings, challenge one another, and agree on a coherent narrative.
- Look through the question summaries. Read them and let them sink in – you need a little undisturbed thinking time. If working you are working as a group, split up the questions and assign each colleague a section.
- Think about what questions you need to answer to fulfil the research brief. Set yourself some clear follow-up questions – if you were your stakeholder(s) what questions would you ask next? What hypotheses do you have about what might be going on?
- Use data tables to answer these questions. Be selective and let your questions dictate what you look at. If your data tables do not answer these questions, think about how they could be restructured so they do answer them.
- Look out for differences by groups of interest – which ones are the most important to pull out?
- Plan your report around answering the research questions. Using your data as evidence, find the ‘story’.
- If you are working in a group, at this stage come together to discuss the overall story and fine tune the narrative. Challenge each other and check that there are no contradictions / there is an agreed message.
Dos
Work out what your overall ‘story’ is before you start to write it down
Consider rebasing some questions so that the story makes more ‘sense’ – often the findings will be more powerful if rebased to be on ‘all respondents’ even if the question was asked only of a subset
Consider what your key variables are – what ‘clever’ things might you be able to do with a handful of questions to really add value
Don’ts
Report questions in question order or feel obliged to report every question
Get waylaid by ‘interesting’ detail – focus on the main findings first
Be scared of reporting the obvious